Smart Energy & Utilities
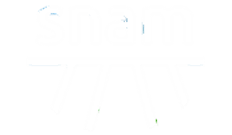
Approach & Solution

Approach
UFG is the quantity of gas remaining from the balance between the gas injected and the gas delivered to end users, net of line-pack variations and of the consumption connected with the operational management of the network.
It has been highlighted that it has a highly variable trend over time and, hence, Snam has carried out several analyses of UFG determinants throughout the years, in order to address actions to reduce such variability.
Continuous discussions and the skills introduced in the measurement sector, which also relate to the chemical / physical analysis of the quality of gas, had identified several variables that influenced the thresholds of the meters.
It was therefore necessary to adopt a more scientific approach, using Advanced Analytics techniques to solve the problem.
Solution
We carried out continuous discussions with Snam on the results of the analyses, which we carried out with the help of machine learning activities, allowed to better understand these phenomena. Hence, we began an industrialisation plan of the models implemented and defined a roadmap to complete the planned cases in 2022.
The architecture defined is based on the Microsoft technology stack: Data Lake Gen2 for data storage, Synapse for data structures, and Azure Machine Learning for the front-end Advanced Analytics.
The user interface was designed as consistent with the choices made for SUMMER, identifying ways of representation appropriate to the market best practices and allowing users to create standard reports independently and autonomously.
Results
Effective interaction with ARERA authority
Reduced Unaccounted for Gas
Improved quality of data analysis
Project value
Innovation
Process performance
Enabling Technologies
AI & Advanced Analytics
Project Team
Data & Analytics
Energy & Utilities
Engineering Interactive